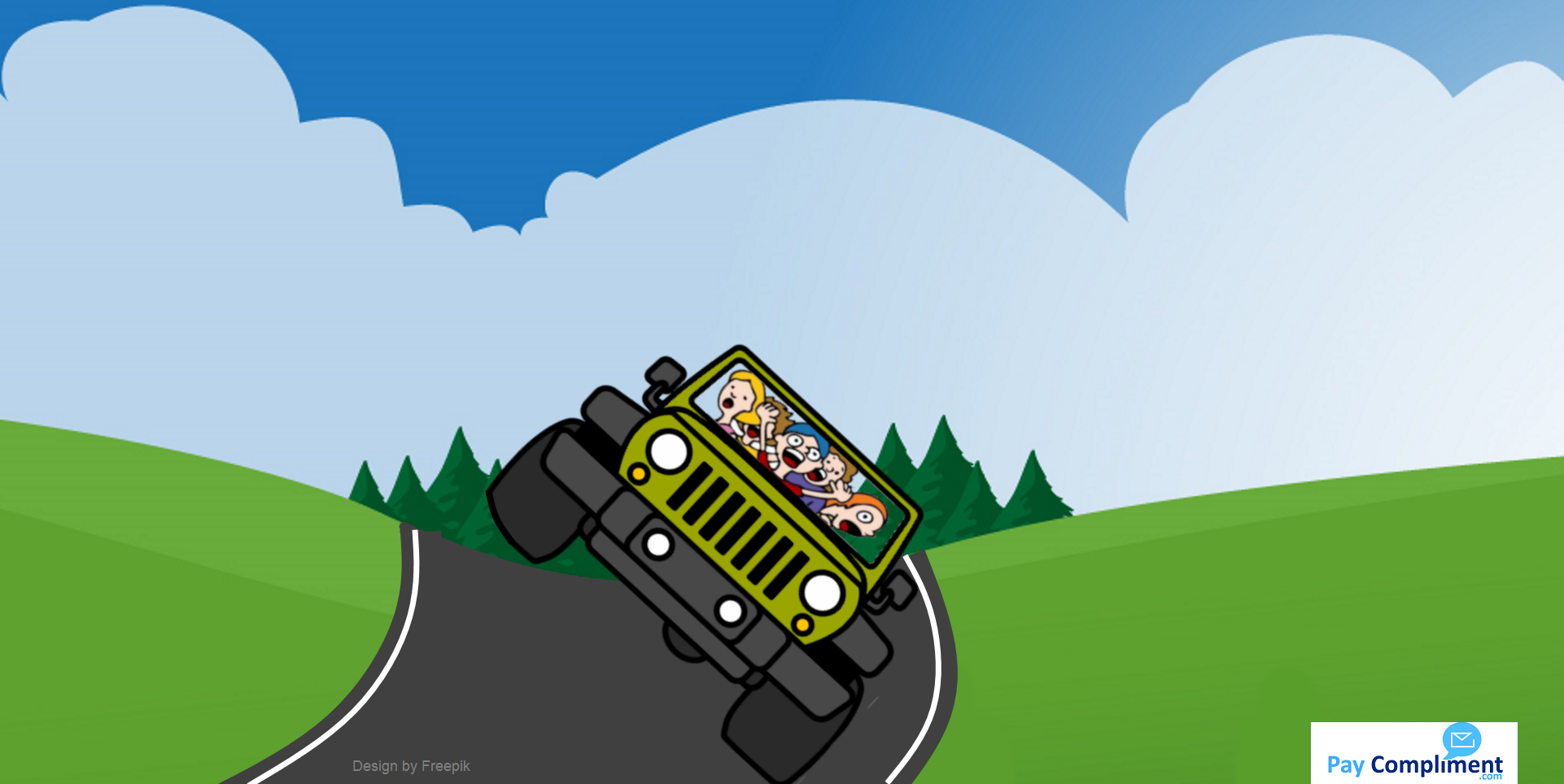
In our last article, we discussed managing without ratings and covered the biases that contribute to idiosyncratic rater effect. The biases we discussed then are only a tiny fraction of the hundreds of social and cognitive biases that will affect you and your organization on the journey to agile performance management.
Today we look at the wider effects of bias and provide some methods to identify and handle bias.
In our game of I-spy, we acknowledge that we are all biased, that we are often unconscious of the biases we hold, and at other times fully aware and even proud to spot them.
Considering that bias can affect every decision we make, we will also see how to better react when we and others spot the bias within.
Who's playing?
If you think you're less biased then others, it's unlikely.
With that mindset, it's more likely that you're a sufferer of illusory superiority bias! That's where you overestimate your own qualities and abilities relative to others.
Take a look at the chart below which studies the association of male gender to career and female gender to family. Note that only 17% of respondents had little to no bias in their association. So 83% of almost 850,000 people assessed showed some bias in this regard.
If you're still unconvinced, and curious about your own leanings, test yourself against this and other social biases using short self-assessment surveys here.
Of course, you may be in the 17% with a neutral position on the gender-career-family association and other social biases. Can you say the same about the spectrum of cognitive biases outlined by Buster Benson in the 2016 Cognitive Bias Codex?
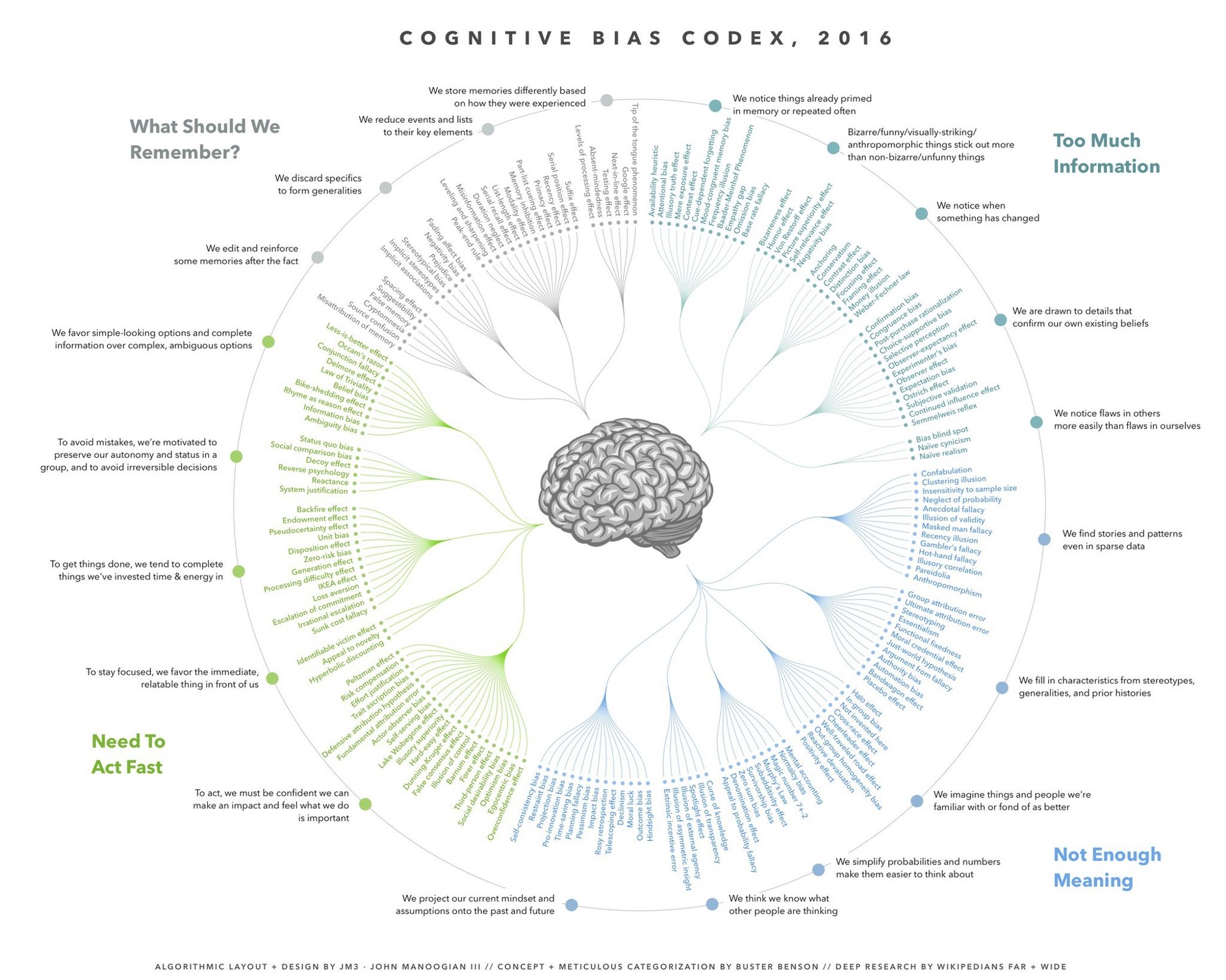
This visualization shows why bias is natural and unavoidable.
It's a mechanism to save the brain time or energy in problem-solving.
It's a shortcut for fast reactions.
Like all shortcuts, bias comes with the tradeoff that we may spend less energy to get a result, but sometimes that result is going to be compromised.
The trick to accommodating bias is to take a risk-based approach. To have the ability to identify those scenarios where decision-making errors are high impact and incidences of bias are occurring with significant regularity.
I-spy: spotting bias
Data is your friend when trying to spot bias. The more data you have at your disposal the easier it becomes to hunt down possible bias.
If there is one single reason for keeping track of coaching conversations and feedback it would have to be to enable data-driven decisions, that are traceable rather than instinctive decisions that are unregulated.
With data-driven decisions, you can ask one critical question to shine a light on likely bias;
"Is it possible to tell a different story using the same information?"
If there are multiple stories that could be told, then bias at some level is influencing the story that is being told.
To make good use of data there are 3 types of analysis to help reveal cultural bias and these can be targeted towards the biases that have most negative impact to your culture. They are
- Sentiment Analysis
- Context Analysis
- Organization Network Analysis
Sentiment analysis can identify the way people feel about things. Beyond tallying positive and negative sentiment, this type of analysis can be used to categorize the specific emotional reaction to workplace issues and the workplace environment. Seeing inconsistency in sentiment can help us spot pockets of bias such as a negative ungrateful manager or a sub-culture inside a division or geography. Seeing wholesale undesirable sentiment can help us see bigger culture problems such as bullying, gender bias, and nepotism.
Context analysis identifies the context to which strong feelings relate. Is it a policy, or work practice that is causing the issue or is it environmental and so on? Context analysis helps us understand the hot issues in the workplace from the macro-level to local issues and to identify what is working well and what is not.
Organization network analysis shows the connections people have to others. This is a powerful tool for measuring diversity and inclusions. Being visual, it makes it very easy to spot in-groups and out-groups in large populations, using filters to see connections (or their absence) within the same community filtered by gender, age, seniority, etc.
Data and analytics as critical to understanding and accommodating bias and being proactive in mitigating possible impacts.
Just to underline this point, if you fall on the side of coaching conversations without record keeping, then you have little real possibility of spotting bias in the moment and you have little choice but to react to the impact of it much later down the line.
The rules and keeping score
There are some road rules to mitigate bias.
In most organisations mitigation starts with awareness of bias and comfort to challenge it. Through education, some self -regulation and peer to peer regulation can be achieved at a grassroots level. Open 2-way conversation and seeking mutual agreement (which may require more data and wider perspective) are the results organisations should be looking for when bias rears its head.
John Lewis Jr., global chief diversity officer at The Coca-Cola Company puts it this way “The courage to speak up, challenge others and the status quo is a central behaviour of an inclusive leader and it occurs at three levels: with others, with the system, and with themselves.”
However, after roughly 15 years of wide-scale training in unconscious bias, history shows awareness and self-regulation are not enough to deal with it.
The new kids on the block to combat bias are the assisting technologies that use Artificial Intelligence (AI) to spot it. These technologies can help bring bias to the forefront and venture into areas where people may be reticent to.
AI technologies can be applied in diverse scenarios such as analysis of job ad's for biased language, analysis of performance assessment for biased observations, analysis of documents, and emails for biased communications to name a few, and of course they are faster and increasingly more effective in spotting bias than humans are.
Interestingly the evolution of AI is Machine Learning where algorithms dynamically adapt as they learn.
Whilst Machine Learning is still in its infancy there are a number of famous examples of machines learning to become too human with the result that they inherit our flaws.
Based on the current state of the art, we think a mix of AI to help spot bias, and education to equip humans with the skills to deal with it is the appropriate formula for success.
Winning against bias
You'll be winning the I-spy of bias if you are able to spot bias as it happens, challenge decisions that are being justified from a perspective of bias and measure that your people do not have negative sentiment to the context that those decisions create.
If you need tools to help mitigate bias and to visualize the state of diversity and inclusion in your workplace, the team at Pay Compliment are standing by to help with a simple platform that provides powerful capabilities.
If you have found this article useful, please share it to help us spread the possibility of transforming the way that organisations manage people.